Lessons for AI Governance from Atoms for Peace, Part 2: Chips for Peace?
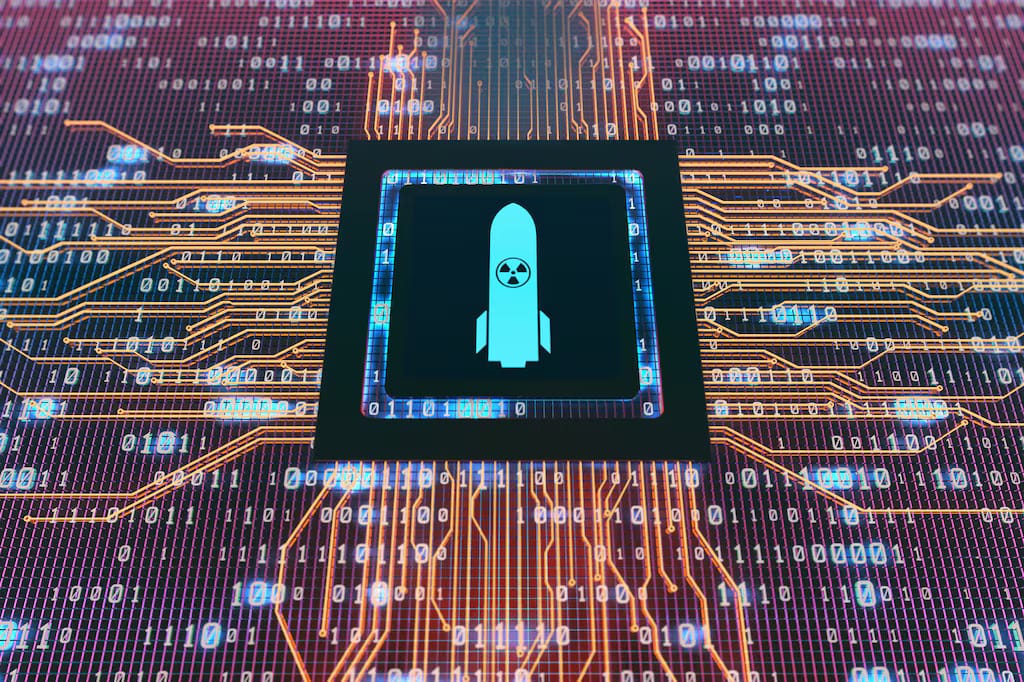
This series of blogposts was co-authored with Dr. Sophia Hatz, Associate Professor (Docent) at the Department of Peace and Conflict Research and the Alva Myrdal Centre (AMC) for Nuclear Disarmament, at Uppsala University. She leads the Working Group on International AI Governance within the AMC.
The need to develop strategy and governance for advanced AI has become urgent, particularly as the catastrophic scale of risks from increasingly advanced AI models become clear, and as destabilizing competitive dynamics emerge. The parallels to nuclear technology and Cold War dynamics are perhaps more apparent now than then ever. But does the logic and strategy of ‘Atoms for Peace’ apply? Our analysis reveals three key challenges.
1. Finding a Grand Bargain for Advanced AI
Although AI is a dual-use technology, identifying a grand bargain is tricky.
In comparison to nuclear weapons, AI’s risks are diverse and diffuse, ranging from smaller and more immediate risks such as algorithmic bias, privacy erosion, and job displacement to broader societal-scale concerns such as robust totalitarianism and loss of human control. Reaching international consensus on which AI risks are the most urgent to mitigate is difficult.
Further, while there is a relatively clear distinction between military and civilian applications of nuclear technology, it is difficult to distinguish between dangerous and beneficial AI. Frontier AI models –general-purpose AI models at the forefront of AI capabilities– offer the closest analogy for nuclear technology as these models could have dangerous capabilities sufficient to pose catastrophic risks[1]. Yet, assessing whether AI model capabilities are approaching critical thresholds is much more complicated than determining whether a country’s nuclear activities are weapons-related or civilian. Dangerous AI capabilities can emerge unexpectedly, often in ways even developers struggle to anticipate or detect. And, unlike nuclear technology, there is no universally recognized “weapons-grade” AI model.
For these reasons, current efforts to identify catastrophically-risk AI models often rely on proxies such as chips, compute or semiconductor supply chains, not necessarily because these are great proxies, but because precise thresholds for catastrophically dangerous AI capabilities are difficult to define.
Identifying “peaceful” uses of AI is equally challenging. Characteristics such as the opacity of AI models, their context-dependent risks, and their vulnerability to manipulation, make it difficult to verify the safety of AI systems. This makes it fundamentally difficult to leverage sharing the tremendous benefits of AI as an incentive.
What would an AI grand bargain actually entail? Under Atoms for Peace, the exchange was clear: access to peaceful nuclear technology in return for international oversight and restrictions on weapons development. In the AI context, a comparable bargain would need to offer meaningful benefits (e.g., access to safe advanced AI models and resources) in exchange for verifiable safety commitments and oversight of high-risk applications. However, the technical challenges outlined above make this difficult to operationalize. Much research and policy work is needed –particularly in areas such as Frontier AI capability evaluations, safety standards and risk assessment– to define the core components of a grand bargain.
2. Leveraging Technological Leads
Atoms for Peace leveraged America's nuclear lead to make credible commitments toward peaceful use. Assuming a coalition of the U.S. and its allies can sustain an advantage in Frontier AI and semiconductor supply chains, can this lead be used to commit to AI risk reduction?
Several commitment problems complicate this endeavor. First, the lead must be substantial enough to give a ‘coalition of the cautious’ sufficient time to make meaningful progress on AI safety. However, recent developments—such as rapid scaling, model distillation and the immanence of AI agents—create uncertainty about both how long the U.S. and its allies can maintain an advantage and how long it will take to develop the necessary technical and governance solutions. Second, the coalition must credibly commit to moving cautiously on Frontier AI development—a challenge made particularly difficult by intensifying U.S.-China competition for AI dominance, which incentivizes a ‘race to the bottom’ in safety. Finally, the coalition must ensure that its risk-reduction efforts do not inadvertently accelerate the development of dangerous AI capabilities. Safety research itself can contribute to capability gains— historically several fields of AI safety research, such as interpretability and preference learning, have driven developments leading to more powerful AI models and this is likely to continue in the future.
These commitment problems reveal important differences from the nuclear precedent. When the U.S. leveraged its nuclear lead through Atoms for Peace, it did so from a position of overwhelming technological advantage that would persist for years. In contrast, the AI landscape is unpredictable, with leadership positions potentially shifting in months rather than decades. Additionally, while nuclear technology development requires massive state-backed infrastructure, AI development is more distributed and often led by private companies with their own competitive incentives.
Addressing these commitment problems will require not only policy levers, but also technical mechanisms that enable verifiable commitments to cautious AI development.
3. Strategic competition
Finally, we need to consider what a ‘Cold War strategy’ would look like in the context of AI. The Cold War emerged post-WWII, featuring stark ideological blocs and existential nuclear fears – a context arguably different from today's more complex geopolitical landscape, fluid alliances, and the specific nature of US-China competition.
Nonetheless, some current strategies echo past containment efforts. Coordinated export controls on high-performance AI chips and manufacturing equipment, for instance, aim to slow AI progress in rival states and consolidate a technological lead for a U.S.-led coalition. While the context differs, the logic mirrors Cold War-era technology containment strategies, where the West restricted access to sensitive military and dual-use technologies to prevent Soviet advancements.
The lesson from Atoms for Peace is cautionary: while it helped establish a U.S.-led nuclear order, it also reinforced Cold War divisions, pushing adversaries toward independent weapons programs and fueling decades of arms racing. Similarly, strict AI export controls could drive states outside the coalition toward self-sufficiency in AI infrastructure. This could result in a bifurcated AI ecosystem with incompatible AI safety standards and lower prospects for broad international coordination.
More dangerously, export controls could reinforce the perception that AI leadership is a zero-sum game, causing restricted states to shift their focus toward AI military applications, and perhaps leading to an AI-arms race. In this scenario, with rival states racing to deploy AI-enhanced capabilities before their adversaries, integrating not-fully-safe advanced AI into military functions ranging from autonomous weapons to strategic decision-support, the result could be greater unpredictability in strategic decision-making, heightened crisis instability and an increased risk of unintended conflict escalation.
Conclusion
The AI-nuclear analogy is valuable not because the technologies are highly similar, but because examining historical precedents can help structure our thinking about the governance of powerful dual-use technologies. Our analysis of Atoms for Peace reveals that applying a similar logic and strategy to advanced AI would face significant challenges.
A more effective strategy could take inspiration not just from Atoms for Peace but from the broader concept of nonproliferation. While nuclear nonproliferation serves a specific function in the context of Atoms for Peace, it has since become a broader international norm and approach to mitigating catastrophic risks from dangerous technologies.
Applying this broader concept, AI nonproliferation would not be narrowly defined as controlling model weights or restricting access to high-performance chips, since these alone do not determine AI’s risk profile. Instead, a broader notion of AI nonproliferation would focus on controlling dangerous AI capabilities—particularly those that disproportionately carry societal-scale or catastrophic risks. As the science of AI capability evaluations improve, and governments become better equipped with empirical evidence, we could move away from relying on proxies.
Further, AI nonproliferation could focus not only on preventing the spread of Frontier AI to actors without adequate safeguards (horizontal proliferation) but also on curbing the unchecked escalation of AI capabilities (vertical proliferation). Atoms for Peace primarily focused on horizontal proliferation, and this focus is maintained in some analogous proposals[2] for AI. Yet, vertical AI proliferation is equally destabilizing—if one actor pushes too far and too fast, AI capabilities could exceed our ability to control them before safety mechanisms are fully developed.
If-then commitments exemplify an approach focused on preventing the vertical proliferation of dangerous AI capabilities. The general idea is that AI developers commit to pause, delay, or limit development and deployment when specific "trip-wire capabilities" are detected through ongoing evaluations. This approach provides some mechanisms to address each of the three key challenges we've identified.
First, if-then commitments do not require broader international agreement on which risks should be prioritized or what the weapons-grade equivalent of advanced AI is ex ante. Instead, AI developers agree that certain situations would require risk mitigation if they came to pass. In avoiding unnecessary restrictions on innovation, if-then commitments support the development of “peaceful” AI. In other words, the grand bargain is that developers maintain freedom to innovate and advance AI capabilities, while accepting pre-defined limits when specific risk thresholds are reached. This also makes a grand bargain more technically feasible and more politically palatable, as it grounds governance in empirical evidence rather than speculative risks.
Second, if-then commitments address some specific commitment problems. For example, by pre-defining specific "trip-wire capabilities" and conditionally committing to risk mitigation, they add credibility to commitments to develop responsibly. Further, if-then commitments could mitigate the "racing" dynamic that otherwise incentivizes cutting corners on safety. If all leading developers were to publicly commit to the same capability triggers, the competitive advantage of rushing ahead diminishes, as reaching certain capability thresholds would activate the pre-committed limitations regardless of who gets there first.
Third, they may help avoid a bifurcated AI ecosystem by creating governance frameworks that can function across geopolitical divides. Unlike export controls focused on hardware, capability-based commitments can be adopted by any developer regardless of nationality, potentially enabling inclusive cooperation around shared safety standards. Constructive relationships could be established with Global South nations based on a shared commitment towards open tech transfers and ensuring a safe and flourishing future with advanced AI. This is necessary in order to avoid repeating the mistakes of the Atoms for Peace, where badly framed measures and inconsistent application of rules caused a loss of trust and led to countries seeking other partners.
While if-then commitments currently exist in some form in responsible scaling policies and other policies voluntarily put forth by leading AI companies, these could eventually be enforced by an international regime, much the way the IAEA and NPT institutionalized nuclear nonproliferation following Atoms for Peace. Eventually, this could lead to agreements on restrictions and safety measures for dangerous AI use cases, including lethal autonomous weapon systems and nuclear weapon command and control.
If-then commitments illustrate that we don’t necessarily need universal agreement on AI risks or for cautious developers to maintain an undisputed lead in order to slow the proliferation of dangerous AI capabilities. However, for AI nonproliferation to work, we do need a robust science of capability evaluation that can reliably detect when AI models approach dangerous thresholds, as well as robust verification mechanisms. Just as voluntary oversight proved insufficient in nuclear governance, AI governance initiatives must incorporate mandatory, transparent evaluations and credible enforcement from the outset. By carefully balancing openness and security, proactively addressing geopolitical mistrust, and designing adaptive institutions, AI governance can avoid repeating historical mistakes. Ultimately, effective AI nonproliferation will depend on clear capability thresholds, rigorous verification, and inclusive international cooperation—all of them areas where AI governance can learn from nuclear governance.
- Anderljung, M., Barnhart, J., Korinek, A., Leung, J., O’Keefe, C., Whittlestone, J., Avin, S., Brundage, M., Bullock, J., Cass-Beggs, D., Chang, B., Collins, T., Fist, T., Hadfield, G., Hayes, A., Ho, L., Hooker, S., Horvitz, E., Kolt, N., Schuett, J., Shavit, Y., Siddarth, D., Trager, R., and Wolf, K. (2023). Frontier AI Regulation: Managing Emerging Risks to Public Safety. ArXiv preprint arXiv:2307.03718v4
- For example, see “Nonproliferation” in Chips for Peace and Superintelligence Strategy.